Neural Networks
Introduction
The dynamics of neural networks and the dynamics of classical spin systems
are strikingly similar in several respects. The storage of information
(firing patterns) or of stimulus-response schemes in neural networks,
for instance, can formally be described as the construction of attractors
in the dynamics of spin systems. This construction is achieved by giving
suitable values to the exchange couplings between the spins, which take the role of
synapses in neural networks. The excitatory or inhibitory nature of synapses
is in this construction corresponds to a ferromagnetic or anti ferromagnetic
nature of the spin-spin interaction. The search for suitable values of synaptic
interactions in a neural net can be done iteratively through a process of
learning or training.
In the course of the last ten years or so, we have adressed questions
concerned with the storage capacity of neural networks, questions related
to neural code (low-activity and hierarchically organized patterns), with
the storage and representation of sequences, with analog or graded-response
neuron systems, with learning algorithms, with preprocessing in feed-forward
systems, with the role of dream-sleep (unlearning), and more.
Very recently we have used neural network type modelling to investigate mechanisms
that might underly the process of cell reprogramming.
Recent talks and a list of publications can be found below.
Recent Talks
-
Neural Networks - From Brains to Gene Regulation (pdf)
Talk presented at a KCL Taster Course (2009)
-
From Neurons to Brains - From Societies of Brains to Brains of Societies (pdf)
Talk presented at: (i) Summer-Academy of the Studienstiftung des Deutschen Volkes, St. Johann
(Sept 1993) (ii) Workshop Human Boundaries, FESt Heidelberg (Feb 2009);
(iii) KCL Taster Day (June 2013); (iv) KCL Department of Mathematics Cumberland Lodge
Student Weekend Conference, Cumberland Lodge (Feb 2014); (v) Workshop Sustainable
Development, Deutsche Bank AG, London (Oct 2014); (vi) ZiF Public Evening Lecture, German
title: Gehirn und Gesellschaft - Eine Analogie, ZiF Bielefeld (Dec 2019)
Video here.
-
Learning with Incomplete Information (pdf)
Talk presented at the Bernstein Symposion on Object Localization,
Herrsching bei München (2007)
-
Tales from the Classroom: no, yes, yes, no, yes,... (pdf)
Talk presented at the Mathematics Teachers' Conference, King's College,
London (2005)
-
Representation and processing of novel stimuli by an associative
neural network (pdf)
Talk presented at the workshop
"Statistical Physics of Molecular and Cell Biological Systems and
Networks" of the ESF SPHINX programmme, Heidelberg (2004)
Publications
I. Books
- Adaptivity and Learning – An Interdisciplinary Debate,
R. Kühn, R. Menzel, W. Menzel, U. Ratsch, M.M. Richter, and I.O. Stamatescu (Eds.),
(Springer, Berlin, 2003)
(Amazon)
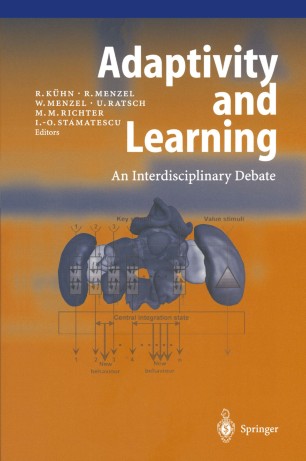
-
Theory of Neural Information Processing Systems,
(Oxford Univ Press, Oxford, 2005)
(Amazon)
II. Papers
Cell Reprogramming Modelled as Transitions in a Hierarchy of Cell Cycles
Ryan Hannam, Alessia Annibale and Reimer Kühn
preprint arXiv:1612.08064 (2016) (pdf);
J. Phys. A 50 425601 (23pp) (2017)(pdf);
(included in the
J Phys A Highlights-of-2017
collection)
We construct a model of cell reprogramming (the conversion of fully differentiated cells to a state of pluripotency, known as induced pluripotent stem cells, or iPSCs) which builds on key elements of cell biology viz. cell cycles and cell lineages. Although reprogramming has been demonstrated experimentally, much of the underlying processes governing cell fate decisions remain unknown. This work aims to bridge this gap by modelling cell types as a set of hierarchically related dynamical attractors representing cell cycles. Stages of the cell cycle are characterised by the configuration of gene expression levels, and reprogramming corresponds to triggering transitions between such configurations. Two mechanisms were found for reprogramming in a two level hierarchy: cycle specific perturbations and a noise induced switching. The former corresponds to a directed perturbation that induces a transition into a cycle-state of a different cell type in the potency hierarchy (mainly a stem cell) whilst the latter is a priori undirected and could be induced, e.g., by a (stochastic) change in the cellular environment. These reprogramming protocols were found to be effective in large regimes of the parameter space and make specific predictions concerning reprogramming dynamics which are broadly in line with experimental findings.
Learning with incomplete information in the Committee Machine
U.M. Bergmann, R. Kühn and und I.O. Stamatescu,
final version (pdf), Biol. Cyb. 99 401-410 (2009)
Learning with incomplete information and the mathematical
structure behind it
R. Kühn and und I.O. Stamatescu,
Biol. Cyb. 97 , 99-112 (2007) .
Representation and Coding of Stimuli by a Population of Neurons I:
The Stationary Regime
R. Kühn,
preprint, submitted to J. Comp. Neurosci.
Learning Structured Data from Unspecific Reinforcement,
M. Biehl, R. Kühn and und I.O. Stamatescu,
J. Phys. A 33 , 6843-6857 (2000)
A Two Step Algorithm for Learning from Unspecific Reinforcement,
R. Kühn and I.O. Stamatescu,
J. Phys. A 32,5749-5762 (1999)
Neural Networks, H. Horner and R. Kühn, in:
Intelligence and Artificial Intelligence, an Interdisciplinary Debate,
edited by U. Ratsch, M. Richter, and I.O. Stamatescu (Springer, Heidelberg 1998) pp
125-161
Averaging and Finite Size Analysis for Disorder: The Hopfield Model
, T. Stiefvater, K. R. Müller, and R. Kühn,
Physica A 232 61-73 (1996)
Multiplicity of Metastable Retrieval Phases in Networks of Multistate
Neurons, S. Bös and R. Kühn, J. Stat. Phys. 76 1495-1504
(1994)
Replica Symmetry Breaking in Attractor Neural Network Models,
H. Steffan and R. Kühn,
Z. Phys. B 95 249-260 (1994)
Storage Capacity of a Two-Layer Perceptron with Fixed Preprocessing
in the First Layer, A. Bethge, R. Kühn, and H. Horner,
J. Phys.
A27, 1929-1937 (1994).
Multifractality in Forgetful Memories, U. Behn, J.L. van Hemmen,
R. Kühn, A. Lange and V.A. Zagrebnov,
Physica D 68, 401-415
(1993)
Optimal Capacities for Graded-Response Perceptrons, D. Bollé,
R. Kühn, and J. van Mourik,
J. Phys. A 26, 3149-3158 (1993)
Statistical Mechanics for Neural Networks with Continuous-Time
Dynamics, R. Kühn, and S. Bös
J. Phys.
A 26, 831-857 (1993).
Statistical Mechanics for Networks of Graded-Response Neurons,
R. Kühn, S. Bös and J.L. van Hemmen,
Phys. Rev. A 43, 2084-2087 (1991)
Increasing the Efficiency of a Neural Network Through Unlearning,
J.L. van Hemmen, L.B. Ioffe, R. Kühn, and M. Vaas, in: Proceedings
of the STATPHYS 17 Conference, edited by C. Tsallis, Physica A163,
386-392 (1990).
Increased Storage Capacity for Hierarchically Structured Information
in a Neural Network of Ising Type, L.B. Ioffe, R. Kühn, and J.L.
van Hemmen, J. Phys. A 22, L1037-L1041 (1989).
Hebbian learning reconsidered: Representation of Static and Dynamic
Objects in Associative Neural Nets, A. Herz, B. Sulzer, R. Kühn
and J.L. van Hemmen, Biol. Cybern. 60, 457-467 (1989).
Complex Temporal Association in Neural Networks, R. Kühn,
J.L. van Hemmen, and U. Riedel, J. Phys. A. 22, 3123--3135 (1989).
The Hebb Rule: Representation of Static and Dynamic Objects in
an Associative Neural Network, A. Herz, B. Sulzer, R. Kühn, and J.L.
van Hemmen, Europhys. Lett. 7, 663-669 (1988)
Forgetful Memories, J.L. van Hemmen, G. Keller, and R.
Kühn, Europhys. Lett. 5, 663-668 (1988)
Temporal Sequences and Chaos in Neural Nets, U. Riedel, R.
Kühn, and J.L. van Hemmen, Phys. Rev. A 38, 1105-1108 (1988).
Martingale Approach to Neural Networks with Hierarchically
Structured Information, S. Bös, R. Kühn, and J.L. van Hemmen, Z. Phys.
B 71, 261-271 (1988)
Nonlinear Neural Networks: II. Information Processing, J.L.
van Hemmen, D. Grensing, A. Huber, and R. Kühn, J. Stat. Phys. 50,
259-293 (1988).
Nonlinear Neural Networks: I. General Theory, J.L. van Hemmen,
D. Grensing. A. Huber, and R. Kühn, J. Stat. Phys. 50, 231-257
(1988)
Storing Patterns in a Spin-Glass Model of Neural Networks Near
Saturation, D. Grensing, R. Kühn, and J.L. van Hemmen, J. Phys. A
20, 2935-2947 (1987)
Nonlinear Neural Networks, J.L. van Hemmen and R. Kühn,
Phys. Rev. Lett. 57, 913-916 (1986)